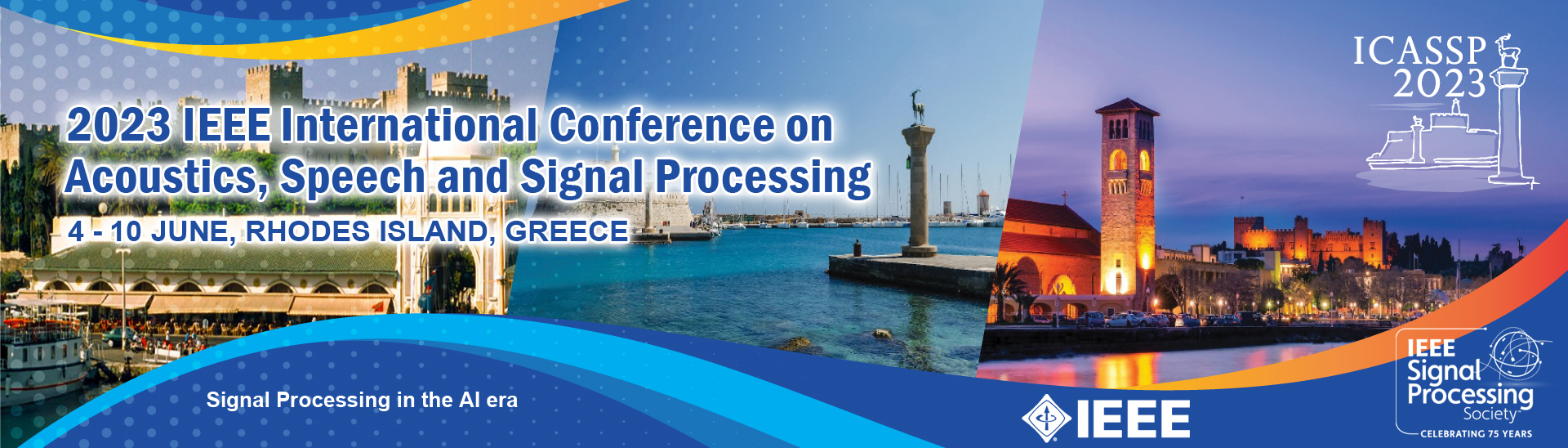
Perspective Talks Speakers
Tuesday (June 06)
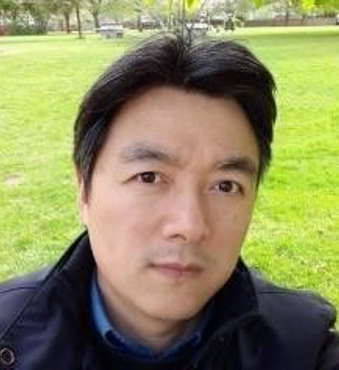
Wenwu Wang
University of Surrey
Abstract:
Cross modal generation of audio and texts has emerged as an important research area in audio signal processing and natural language processing. Audio-to-text generation, also known as automated audio captioning, aims to provide a meaningful language description of the audio content for an audio clip. This can be used for assisting the hearing-impaired to understand environmental sounds, facilitating retrieval of multimedia content, and analyzing sounds for security surveillance. Text-to-audio generation aims to produce an audio clip based on a text prompt which is a language description of the audio content to be generated. This can be used as sound synthesis tools for film making, game design, virtual reality/metaverse, digital media, and digital assistants for text understanding by the visually impaired. To achieve cross modal audio-text generation, it is essential to comprehend the audio events and scenes within an audio clip, as well as interpret the textual information presented in natural language. Additionally, learning the mapping and alignment of these two streams of information is crucial. Exciting developments have recently emerged in the field of automated audio-text cross modal generation. In this talk, we will give an introduction of this field, including problem description, potential applications, datasets, open challenges, recent technical progresses, and possible future research directions.
Biography:
Wenwu Wang is a Professor in Signal Processing and Machine Learning, and a Co-Director of the Machine Audition Lab within the Centre for Vision Speech and Signal Processing, University of Surrey, UK. He is also an AI Fellow at the Surrey Institute for People Centred Artificial Intelligence. His current research interests include signal processing, machine learning and perception, artificial intelligence, machine audition (listening), and statistical anomaly detection. He has (co)-authored over 300 papers in these areas. He has been involved as Principal or Co-Investigator in more than 30 research projects, funded by UK and EU research councils, and industry (e.g. BBC, NPL, Samsung, Tencent, Huawei, Saab, Atlas, and Kaon).
He is a (co-)author or (co-)recipient of over 15 awards including the 2022 IEEE Signal Processing Society Young Author Best Paper Award, ICAUS 2021 Best Paper Award, DCASE 2020 Judge’s Award, DCASE 2019 and 2020 Reproducible System Award, LVA/ICA 2018 Best Student Paper Award, FSDM 2016 Best Oral Presentation, and Dstl Challenge 2012 Best Solution Award.
He is a Senior Area Editor for IEEE Transactions on Signal Processing, an Associate Editor for IEEE/ACM Transactions on Audio Speech and Language Processing, an Associate Editor for (Nature) Scientific Report, and a Specialty Editor in Chief of Frontier in Signal Processing. He is a Board Member of IEEE Signal Processing Society (SPS) Technical Directions Board, the elected Chair of IEEE SPS Machine Learning for Signal Processing Technical Committee, the Vice Chair of the EURASIP Technical Area Committee on Acoustic Speech and Music Signal Processing, an elected Member of the IEEE SPS Signal Processing Theory and Methods Technical Committee, and an elected Member of the International Steering Committee of Latent Variable Analysis and Signal Separation. He was a Satellite Workshop Co-Chair for INTERSPEECH 2022, a Publication Co-Chair for IEEE ICASSP 2019, Local Arrangement Co-Chair of IEEE MLSP 2013, and Publicity Co-Chair of IEEE SSP 2009.
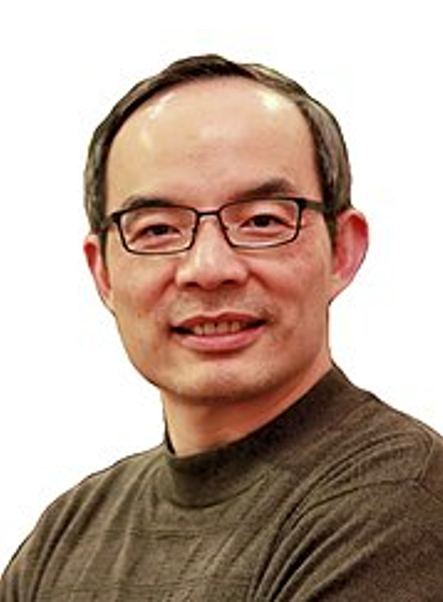
Xuedong Huang
Microsoft AI
Abstract:
We have been on a quest to advance AI beyond existing techniques, by taking a more holistic, human-centric approach to learning and understanding. We view the relationship among three attributes of human cognition: monolingual text (X), audio or visual sensory signals, (Y) and multilingual (Z) to be essential to derive from the intersection: what we call XYZ-code, a joint representation to create more powerful AI that can speak, hear, see, and understand humans better. We believe XYZ-code will enable us to fulfill our long-term vision: cross-domain transfer learning, spanning modalities and languages. The goal is to have pretrained models that can jointly learn representations to support a broad range of downstream AI tasks, much in the way humans do today. The AI foundation models provided us with strong signals toward our more ambitious aspiration to produce a leap in AI capabilities, achieving multisensory and multilingual learning that is closer in line with how humans learn and understand. The journey of achieving such integrative AI also must be grounded with external knowledge sources in the downstream AI tasks.
Biography:
Xuedong Huang is a Microsoft Technical Fellow and served as Microsoft’s Azure AI Chief Technology Officer.
In 1993, Huang left Carnegie Mellon University to found Microsoft’s speech technology group. Huang has been leading Microsoft’s spoken language efforts for over 30 years. In addition to bringing speech to the mass market, Huang led Microsoft in achieving several historical human parity milestones in speech recognition, machine translation, and computer vision. He is best known for leading Microsoft Azure Cognitive Services from its inception, including Computer Speech, Computer Vision, Natural Language, and OpenAI, to making Azure AI an industrial AI platform serving billions customers worldwide.
Huang is an IEEE fellow (2000) and ACM fellow (2017). Huang was elected as a member of the National Academy of Engineering (2023) and the American Academy of Arts and Sciences (2023).
Huang received his BS, MS, and PhD from Hunan University (1982), Tsinghua University (1984) and the University of Edinburgh (1989) respectively.
Wednesday (June 07)
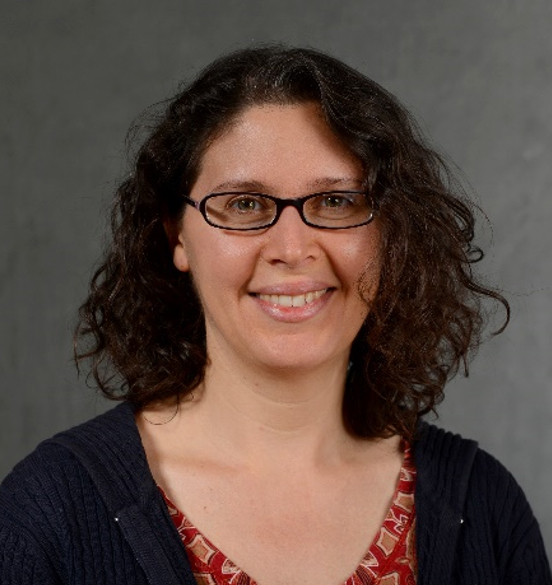
Karen Livescu
Toyota Technological Institute at Chicago
Abstract:
Sign languages are used by millions of deaf and hard of hearing individuals around the world. Research on sign language video processing is needed to make all of the technologies that are now available for spoken and written languages available also for sign languages. Sign languages are under-resourced and unwritten, so research in this area shares many of the same challenges faced by research on other low-resource languages. However, there are also sign language-specific challenges, such as the difficulty of analyzing fast, coarticulated body pose changes. Sign languages also include certain linguistic properties that are specific to this modality.
There has been some encouraging progress, including on tasks like isolated sign recognition and sign-to-written language translation. However, the current state of the art is far from handling arbitrary linguistic domains and visual environments. This talk will provide a perspective on research in this area, including work in my group and others aimed at a broad range of real-world domains and conditions. Along the way, I will present recently collected datasets as well as technical strategies that have been developed for handling the challenges of natural sign language video.
Bio:
Karen Livescu is a Professor at Toyota Technological Institute at Chicago (TTI-Chicago). She completed her PhD in electrical engineering and computer science at Massachusetts Institute of Technology (MIT) in 2005 and her Bachelor’s degree in physics at Princeton University in 1996. Her main research interests are in speech and language processing, as well as related problems in machine learning. Her recent work includes self-supervised representation learning, spoken language understanding, acoustic word embeddings, visually grounded speech and language models, and automatic sign language processing.
Dr. Livescu is a 2021 IEEE SPS Distinguished Lecturer and an Associate Editor for IEEE TPAMI, and has previously served as Associate Editor for IEEE/ACM TASLP and IEEE OJSP. She has also previously served as a member of the IEEE Speech and Language Processing Technical Committee and as a Technical Co-Chair of ASRU. Outside of the IEEE, she is an ISCA Fellow and has recently served as Technical Program Co-Chair for Interspeech (2022); Program Co-Chair for ICLR (2019); Associate Editor for TACL (2021-present); and Area Chair for a number of speech, machine learning, and NLP conferences.
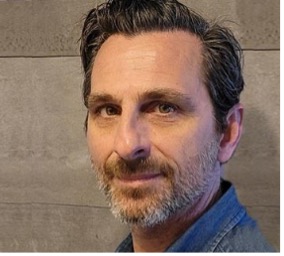
Spyros Raptis
Innoetics / Samsung Electronics Hellas
Abstract:
Powered by the recent advances in AI-based representation and generation, text-to-speech technology has reached unprecedented levels in quality and flexibility.
Self-supervised learning techniques have provided ways to formulate efficient latent spaces claiming more control over different qualities of the generated speech, zero-shot training allowed matching the characteristics of unseen speakers, and efficient prior networks contributed to disentangling content, speaker, emotion and other dimensions of speech.
These developments have boosted existing application areas but also allowed tackling new ones that previously seemed much more distant. We’ll discuss some of the recent advances in specific areas in the field, including our team’s work on multi-speaker, multi-/cross-lingual, expressive and controllable TTS, on synthesized singing, as well as on automatic synthetic speech evaluation. We’ll also look into cloning existing speakers as well as generating novel ones.
Finally, we’ll touch on the valid concerns that such unprecedented technical capabilities raise. Voice is a key element of one’s identity and although such technologies hold great promise for useful applications, at the same time they have a potential for abuse, thus raising ethical and intellectual property questions, both in the context of the creative industries and in our everyday lives.
Biography:
Spyros Raptis is the Head of Text-to-Speech R&D at Samsung Electronics Hellas. Before joining Samsung he was the Director of the Institute for Language and Speech Processing (ILSP), Vice president of the Board of the “Athena” Research Center, and co-founder of the INNOETICS startup company which was acquired by Samsung Electronics.
He holds a Diploma and a PhD on computational intelligence and robotics from the National Technical University of Athens, Greece.
He has coordinated various national and European R&D projects in the broader field of Human-Computer Interaction, and he has led the ILSP TTS team who developed award-winning speech synthesis technologies at international competitions.
He is the co-author of more than 60 publications in scientific books, journals and international conferences in the areas of speech processing, computational intelligence, and music technology. He has taught speech and language processing at pre- and post-graduate levels.
His research interests include speech analysis, modeling and generation, voice assistants and speech-enabled applications and services, tools for accessibility etc.
Thursday (June 08)
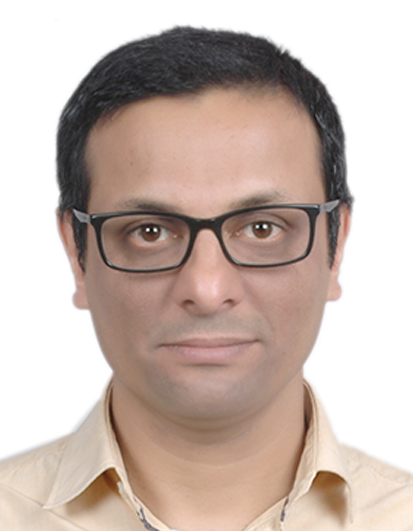
Chandra Murthy
Indian Institute of Science
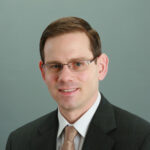
Anthony Vetro
Mitsubishi Electric Research Labs